英语翻译We now define the learning problem and the user-interaction model more generally.At each round t,our algorithm presents a ranking yt from a corpus xt ∈ X of candidate documents1.We assume that the user acts (approximately) rationa
来源:学生作业帮助网 编辑:作业帮 时间:2024/07/11 18:56:05
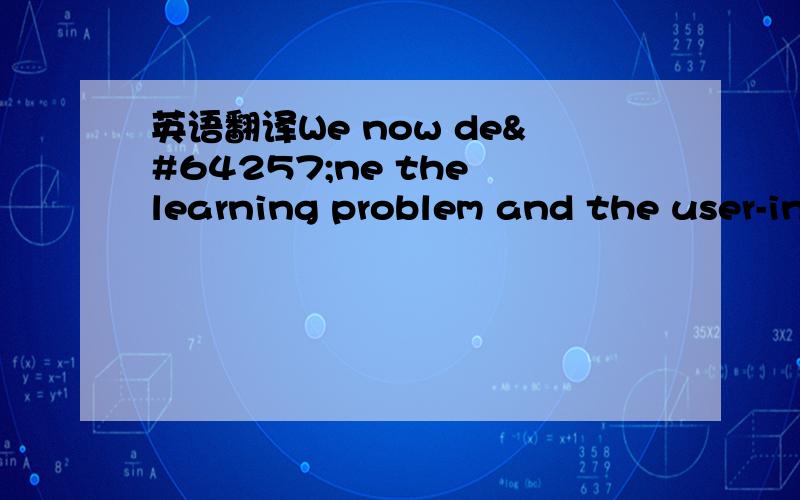
英语翻译We now define the learning problem and the user-interaction model more generally.At each round t,our algorithm presents a ranking yt from a corpus xt ∈ X of candidate documents1.We assume that the user acts (approximately) rationa
英语翻译
We now define the learning problem and the user-interaction model more generally.At each round t,our algorithm presents a ranking yt from a corpus xt ∈ X of candidate documents1.We assume that the user acts (approximately) rational ac- cording to an unknown utility function U(xt,yt) that models both relevance of the documents as well as their dependen- cies (e.g.redundancy).In the context of such a utility function,we can interpret the user feedback as a preference between rankings.This type of preference feedback over multiple rounds t is the input for our learning model.Given the set of candidate documents xt,the optimal ranking is denoted by
y∗ t := arg max y∈Y U(xt,y).(1)
Since the user’s utility function U(xt,y) is unknown,this optimal ranking y∗ t cannot be computed.The goal of the learning algorithm is to predict rankings with utility close to that of y∗ t .Note,however,that the user feedback does not even give the optimal y∗ t to the algorithm (as in traditional supervised learning),but only the user feedback ranking y¯t is observed.To analyze the learning algorithms in the sub- sequent sections,we refer to any feedback that satisfies the following inequality as strictly α-informative feedback:
U(xt,y¯t) − U(xt,yt) ≥ α(U(xt,y∗ t ) − U(xt,yt)).(2)
英语翻译We now define the learning problem and the user-interaction model more generally.At each round t,our algorithm presents a ranking yt from a corpus xt ∈ X of candidate documents1.We assume that the user acts (approximately) rationa
供参考:
现在,我们来从更加广义的角度来定义“学习”问题以及用户交互模型.按照我们的算法,在每轮t之后,对于某个待评估文档个体xt∈ X,可以获取一个(用户)评分yt.假设用户(对文档的)反应(大体上)可表述为一个未知的实用函数U(xt,yt),该函数与文档本身相关(即xt)相关,也与它们的依存度(即冗余度)相关.按照这样函数的表述而言,我们可以将用户(对文档的)反馈视为评分间的传递参数.这种类型的参数反馈经过多轮演绎之后就会形成我们的学习模型的输入.假定备选文档集合为xt,则理想的评分表达式为:
由于用户的实用函数U(xt,yt)未知,因此理想评分y*t是无法计算的.我们研究学习算法的目的是要找出一个尽量接近于y*t的使用函数,用以预测评分.不过请注意,根据用户反馈是不能按本算法(如同传统的授课式教学)得出理想y*t的,而是只能观测到用户反馈评分y-t.为了在后续章节中便于分析学习算法,我们可以将满足下列不等式的反馈作为严格α信息反馈:
我们现在定义的学习问题和用户交互模型更普遍。在每一轮t,我们的算法提出了一种从语料库xt排名次的documents1∈X候选人。我们假设用户行为(大约)理性的ac -连接到一个未知的效用函数U(xt,刘日东),模拟了相关性的文献资料以及他们的dependen -私事(如冗余)。在这样特殊的效用函数,我们可以解释用户反馈作为一个偏好之间的排名。这种类型的偏好反馈在多轮t是为我们的学习模型的输入。给定...
全部展开
我们现在定义的学习问题和用户交互模型更普遍。在每一轮t,我们的算法提出了一种从语料库xt排名次的documents1∈X候选人。我们假设用户行为(大约)理性的ac -连接到一个未知的效用函数U(xt,刘日东),模拟了相关性的文献资料以及他们的dependen -私事(如冗余)。在这样特殊的效用函数,我们可以解释用户反馈作为一个偏好之间的排名。这种类型的偏好反馈在多轮t是为我们的学习模型的输入。给定的一组候选文档xt,优化排名是用y∗t:= y∈arg马克斯y U(xt,y)。(1)从用户的效用函数U(xt,y)是未知的,这个优化排名y∗t无法计算。学习算法的目标是预测排名与效用接近y∗t。但是请注意,用户反馈甚至不能给予最优y∗t的算法(如传统的监督学习),但只有用户反馈排名y¯t是观察。分析学习算法在子——后继章节,我们将任何反馈,满足下列不等式作为严格α-informative反馈:U(xt,y¯t)−U(xt,刘日东)≥α(U(xt,y∗t)−U(xt,刘日东))。(2)
收起